Reframing perspectives on migration projections
The study "Migration flows by age, sex, and educational attainment" enhances our understanding of global migration patterns. Co-authored by Dr. Dilek Yildiz from the PREMIUM_EU team and the International Institute for Applied Systems Analysis, the working paper incorporates critical data into the Wittgenstein Centre's global population projections, focusing on the aspects of age, sex, and educational attainment. This research presents a comprehensive and refined approach to analyzing and predicting migration trends, which is essential not just for PREMIUM’s continued projections but also for future demographic studies and policy-making.
The study breaks new ground by using a combination of random forest models and extensive demographic data. This sophisticated approach allows for a nuanced analysis of migration trends across 183 countries.
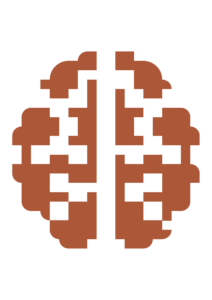
Key findings: a breakdown
- Age dynamics: Young working-age groups (20-30 years) show the highest migration proportions with sizeable differentials in the level and peak age across countries, an important insight for workforce planning.
- Gender perspectives: The study reveals distinct patterns in migration based on sex, indicating unique migration trends for each gender at different life stages, offering a deeper understanding of gender-specific migration issues.
- Educational impact: A noteworthy finding is the varying migration trends based on educational attainment, delving into the 'brain drain' phenomenon in particular. The study identifies significant variations in the educational attainment of migrants across different countries, underscoring the diverse nature of migration flows.
- Economic and demographic indicators: Predictably, GDP, HDI education distribution are crucial in shaping migration patterns, as revealed by the study's analysis.
Methodological rigor
The study's strength lies in its rigorous methodology. Utilizing data spanning from 1990 to 2020, it employs random forest models (Breiman 2001) - a form of advanced machine learning algorithm - to predict migration proportions with unprecedented precision. This technique involves analyzing vast datasets to identify patterns and correlations that traditional statistical methods might overlook.
The researchers meticulously calibrated these models to account for the complexities of migration, considering factors such as economic conditions, human development, and size of diaspora, which are influential in migration decisions. This robust approach not only enhances the accuracy of migration projections but also provides valuable insights into the underlying drivers of migration patterns. The integration of these advanced statistical techniques marks a significant step forward in the field of demographic studies, offering a more nuanced and comprehensive understanding of migration flows.
Conclusions and forward path
This research is a significant step forward for the PREMIUM_EU project, offering a comprehensive framework that melds age, sex, and education in migration projections, revealing that migration predictions can be significantly refined by considering these factors. It opens avenues for more informed policy-making and societal planning, tailored to the evolving dynamics of global migration, which is exactly how the PREMIUM_EU project will culminate.